- New measures: Variants of the c statistics for survival, reclassification tables, NR, IDI
- Decision analytics measures : “decision curves” by making decisions based on model predictions
- Validation in fully independent, external data is the best way to compare the performance of a model with and without a new marker
- Nagelkerke’s R2 test for logarithm predictions is based on the difference in the -2 log likelihood of a model and a model with one or more predictors
- Scaled Brier Score very similar to the Pearson’s R2 statistic
- C statistic is related to Somer’s D
- A popular extension of the C statistics with censored data can be obtained by ignoring the pairs that cannot be ordered
- Addition to the c statistics is the discriminatory slope
- Smoothing technique: Loess algorithm
- Recalibration framework proposed by Cox
Cook proposed a reclassification test as a variant of the Hosmer-Lemeshow statistic within the reclassified categories leading to the CH2 statistic (idea extended by Pencia et al by conditioning on the outcome) - Net classification Improvement (NRI)
- Youden index implies weighting by the non-events odds
- Documentation of decision-curve analysis can be found at: http://www.decisioncurveanalysis.org
- Cook’s reclassification test
- Reclassification can be assessed using a scatter plot before and after change in the model.
- Use design library to generate good plots in R
- Recalibration parameters as proposed by Cox (intercept and calibration slope) are more informative
- Key information for comparing performances of 2 models is contained in the margins of the reclassification tables
Assessing the Performance of Prediction Models
Original by E.W.Steyberg, A.J.Vickers et al, 2010, 11 pages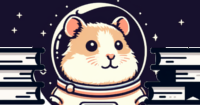

Latest Hamster Notes
- Start with Why posted in Management
- 4 steps to optimise product value posted in Agile Management
- 5 paradoxes of digital business leadership posted in Agile
- Product Vision, 10 tips posted in Agile
- Progress in adopting the Principles for effective risk data aggregation and risk reporting 2023 posted in Credit risk Finance
- Leadership styles by ChatGPT posted in Management
- Gen AI round table posted in Machine Learning
- Leadership in fully remote teams posted in Management
- Leadership for the reluctant leader posted in Management
- Corporate Credit Risk Modelling and the Macroeconomy posted in Finance