- A common and simple approach to evaluate model is to regress predicted vs observed values (or vice versa) and compare slope and intercept parameters against the 1:1 line
- Present mathematical evidence that evaluating the model based on the regression of predicted (y-axis) (PO) and observed (x-axis) leads to erroneous estimates of the slope and intercept
- Observed (y-axis) vs predicted (x-axis) (OP) should be used
- There is no consensus on which variable should be placed in each axis to present the results
- The scatter plot of predicted and observed values (and vice versa) is still the most frequently used approach
- R^2 remains the same for PO or OP
- The slope and the intercept must be calculated only by regressing OP data because in that case the residuals are independent of the predicted value (while they are independent of the observed values in PO)
- Recommends to test the significance of slope =1 and intercept = 0
- The difference between the two slopes increases as R^2 decreases
- The lack of symmetry in the computation of several parameters when regression OP or PO has been noted by several authors (Kobayashi and Salam, 2000; Gauch et al, 2003; Mitchell, 1997)
- The root mean square error (RMSE) should not be applied for the regression of OP data, use the root mean squared deviation instead (RMSD)
- The deviation of each predicted values should be made against the 1:1 slope and not against the regression line for either OP or PO
- RMSE will always be smaller than the RMSD and is thus an underestimation of the real error
How to evaluate models: Observed vs. predicted or predicted vs. observed?
Original by G. Pineiro, S. Perelman, J.P Guerschman, J. Paruelo, 2018, 7 pages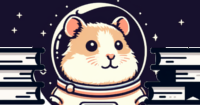

Latest Hamster Notes
- Start with Why posted in Management
- 4 steps to optimise product value posted in Agile Management
- 5 paradoxes of digital business leadership posted in Agile
- Product Vision, 10 tips posted in Agile
- Progress in adopting the Principles for effective risk data aggregation and risk reporting 2023 posted in Credit risk Finance
- Leadership styles by ChatGPT posted in Management
- Gen AI round table posted in Machine Learning
- Leadership in fully remote teams posted in Management
- Leadership for the reluctant leader posted in Management
- Corporate Credit Risk Modelling and the Macroeconomy posted in Finance